ML a subset of AI – For eCommerce Professionals to Understand
How thoroughly and clearly you understand the concept behind AI and ML, their influence in our daily life, and how important they are for your business. Let’s start from the beginning, AI stands for Artificial Intelligence while ML stands for Machine Learning.
Even though our two keywords in today’s article became a mainstream topic in the last ten years due to the high volume of data globally stored and processed, the idea of AI and ML started way back in the past.
Luckily, my team understood the importance of AI and ML, and we implemented them in our business, but I will tell you that story later on. Let’s clarify first, what’s older AI or ML?
The question that arose in the first half of the last century was “Can computers think?”
But long before that time, philosophers and mathematicians speculated about the idea of artificial beings, mechanical people, and other machines. However, they were unsure whether these creatures already existed in the past or they represented the future. The specific idea that human thinking can be implemented into machines was born in the 1700s by great thinkers. From the initial idea to the present day, we follow the daily progress of the artificial industry and all the inventions that have helped the human race so far.
Here is the AI history of the early 20th century summed up in one sentence: many people knew, even before it was proven, that computers could have human reasoning, but at the time, technology was poor, and the costs of using computers were too high, therefore the experiments were postponed.
The main issue was a lack of computer storage and the inability to process data fast enough. All these technical obstacles affected the inflows of funds. Just as government and private funding in research have fluctuated, so has public hype. Experts were on a roller coaster of success and setbacks.
AI was established as a term after a series of research that started in the ’50s, focused on problem-solving and decision-making. The first significant implementation of AI happened in the ’60s by the US Department of Defense. It started as a process where computers were trained to mimic human reasoning.
The moment in which artificial intelligence successfully opposes human intelligence for the first time was the chess match between the world chess champion, Garry Kasparov, and the computer, Deep Blue. In 1997, the man was defeated.
Let’s come back to the present century and present the official definition of artificial intelligence. AI is the ability of a computer or a robot controlled by a computer to do tasks that are usually done by humans because they require human intelligence and discernment.
The first thing that comes to a person’s mind who isn’t well-versed in computer science when hearing the term Artificial Intelligence is, of course, robots. AI has evolved over the years but still, it does not have the abilities presented in the movies where robots are taking over the world.
Where are we now?
Today, we certainly know that computers can mimic human intelligence. AI is integrated into contemporary person’s day-to-day life so well that we are unaware of how helpful and handy some of the AI inventions are.
I will list my top three AI conveniences:
- In 2002 i-Robot Roomba was released. 20 years later, it offers more than vacuuming while avoiding obstacles, it even cleans out itself.
- Virtual Assistant – No matter what brand you’re a fan of – Apple released Siri (2011), Microsoft released Cortana (2014), Amazon released Alexa (2014), Google released Google Home (2016), and Samsung released Bixby (2018).
- Social Media – famous algorithm that everyone talks about and relies on is actually Artificial Intelligence. Bearing in mind that the position of Social Media Manager did not even exist 20 years ago, we are all witnesses to the power of Artificial Intelligence.
As you could realize, AI adds intelligence to existing products. We did have vacuums and phones before the 2000s. In addition to engaging Artificial Intelligence to perfect frequent, high-volume, and computerized tasks, it plays the main role in improving existing technologies.
The above-mentioned, algorithms, along with the computers’ capabilities to collect large amounts of data and process them at a high speed, allow the software to learn automatically. Lately, the role of data is greater than ever before. As far as business is concerned, the best data can give you a comparative advantage in the market. All you have to do is apply AI to get the most out of it. Just as a self-learning algorithm can play chess, it can teach itself what product would be the best to recommend next to your eCommerce clients.
Officially there are 4 types of AI:
- reactive machines
- limited memory
- theory of mind
- self-awareness
Reactive Machines is the oldest category of AI that performs basic operations. The architecture of those machines is simplest, and they don’t perform any learning, do not store inputs, and cannot evolve. One of the famous inventions of this category is Blue Deep, the machine that beat human intelligence for the first time.
Limited memory is a category that offers much more, it has the ability to store the incoming data, as well as the data about any made decision or action. Here is where ML (Machine Learning) has been introduced.
The last two AI categories, Theory of Mind and Self-Aware, are yet to be achieved, so far those are only theories. The upcoming phases of AI are expected to interact with human emotions and thoughts. The ultimate goal of artificial intelligence operations is to create a self-aware machine. Here, we can again mention society’s perception of AI and how it instills both immense amounts of hope and fear at once, which is not a meaningless feeling since the ultimate goal is to create machines that have consciousness at the human level, with similar desires and emotions as humans.
ML – a subset of AI that trains a machine how to learn
ML subset is specifically based on the hypothesis that machines are able to learn and adjust through experience. Its ultimate goal is to learn from the input and data collected through experience in order to give accurate output. There is a list of differences in the abilities of AI and ML for your better understanding.
ML has its own subtypes, there are three main categories that cover a range of complexity:
- supervised learning
- unsupervised learning
- reinforcement learning
These categories differ in the level of human involvement in the processes. Let’s jump into the use right away. Supervised Learning processes require human monitoring and dataset inputs. It applies in processes of image recognition, media recommendation systems, predictive analytics, and spam detector. Unsupervised Learning requires no human involvement in the processes and it is applicable in the medical field to detect anomalies and perform medical imaging but it can also determine customer segments in marketing data. Reinforcement Learning is the most complex category due to the lack of the dataset provided for machine training. Instead, a machine learns on a basis of positive and negative rewards. Reinforcement Learning operations are involved in self-improving industrial robots, automated stock trading, advanced recommendation engines, and bid optimization for maximizing ad spend.
Why is ML important?
Let’s start again with my personal ML favorites, which are Maps and Navigations. In 2022, it is highly unlikely to get lost as long as you have a device with service (data) to be able to access either Waze, Google Maps, or Apple Maps. If you were wondering how your maps detect traffic, road barriers, and always suggest a more optimal route as soon as the change in traffic flow appears, that is because of Machine Learning algorithms.
What about the business world? Yes, all the above-mentioned benefits our personal lives, but what is the importance of AI and ML implementation in our business lives?
As already mentioned, the significance of data in today’s business world is crucial. Good data gives you an edge over the competition. As an individual business entity, you would have to be a data specialist to be able to collect, process, analyze, and utilize the data with an aim to increase profit. Luckily, today, there are automated systems that offer their services in every aspect of the business.
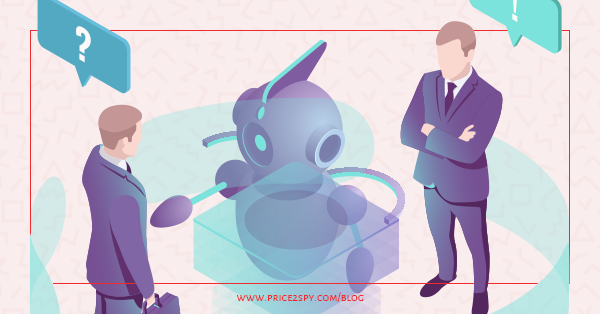
The eCommerce industry is tightly related to the technology industry. Both industries are fast-paced, especially in recent years. With the innovations in Artificial Intelligence (specifically ML), the eCommerce industry managed to improve customer experience, increase sales, and perfect product recommendations for leads. This is predominantly thanks to robust and accurate data labeling and iterative model training to meet the target outcomes. It is estimated that by the end of 2025, AI and ML algorithms will control 95% of customer interactions.
The most notable applications of ML in the eCommerce industry are:
- personalized customer experience
- managing supply and demand
- dynamic pricing
- inventory optimization
- review management
- price monitoring
- fraud detection
Do you remember the story from the beginning that I promised to tell you towards the end?
In every business aspect but especially in the eCommerce industry, price monitoring is one of the most important activities if you aim to be on the top of the market. Price monitoring could be your main weapon when it comes to competitor advantage. Whether you are a retailer, a brand, or a distributor, you have to monitor and adjust to market prices in order to stay competitive. Thanks to Artificial Intelligence, there is a whole market of price monitoring software that offers all kinds of bells and whistles.
For you to understand how the Price2Spy team utilized ML, you have to understand the process behind price monitoring. Here are the steps for you to take (or we can do it for you):
- identify your competitors
- identify products you want to monitor
- define monitoring frequency
- gather URLs
- analyze the data
This list covers the process, from your standpoint, but not from the standpoint of the software. Right before price monitoring, the software has to link your product with the products of your competitors to be able to track the prices. This process is called product matching. Again, every software on the market performs this process differently with different levels of accuracy and different approach.
Price2Spy have three ways of how products can be matched:
- automatch (99.5% matching accuracy)
- manual product matching (99.8% matching accuracy)
- hybrid product matching (99.8% matching accuracy)
Depending on your industry and preferences, we will choose the best approach for you. In order to improve our service and to optimize the product matching process, we have conducted 18-month long research to be able to implement the Product Matching via Machine Learning project (Abstract & Example of Product Matching) in June 2020.
Product Matching via Machine Learning is meant primarily for those of you whose products can not be easily matched by unique identifiers (EAN, MPN, UPC, etc.). Therefore, there is an urge for manual product matching. At least that was the case before our project. Nowadays, you can choose Hybrid Automatch via Machine Learning for your company. In this case, hybrid means that ML algorithms and humans are working together. First, ML algorithms crawl through your and your competitors’ sites. After the process of matching is done by the machine, humans do the final check to make sure that all matching suggestions are meaningful to your business.
In case you are not in a position to develop your personal project, it is understandable, those are time-consuming and costly. At least make sure to partner up with companies that have utilized the benefits of AL and ML because only that way you will be able to respond to 2022 eCommerce challenges.
If there are any Machine Learning (ML) enthusiasts among our readers, please check out the following links to familiarize yourself in-depth with our project:
- Product matching via Machine Learning – Abstract
- (Part #1) Product m via Machine Learning – Introduction to the project
- (Part #2) Product matching via Machine Learning – Important decisions to be made
- (Part #3) For ML experts – why is product matching so difficult
- (Part #4) Preparing the ML training set
- (Part #5) ML training Implementation
- (Part #6) Evaluating ML training results
- (Part #7) Product matching via ML: Post-processing
- (Part #8) Product matching via ML: Testing on various industries/languages
- (Part #9) ML does work, but it’s not magic
- (Part #10) Product matching via ML: The results